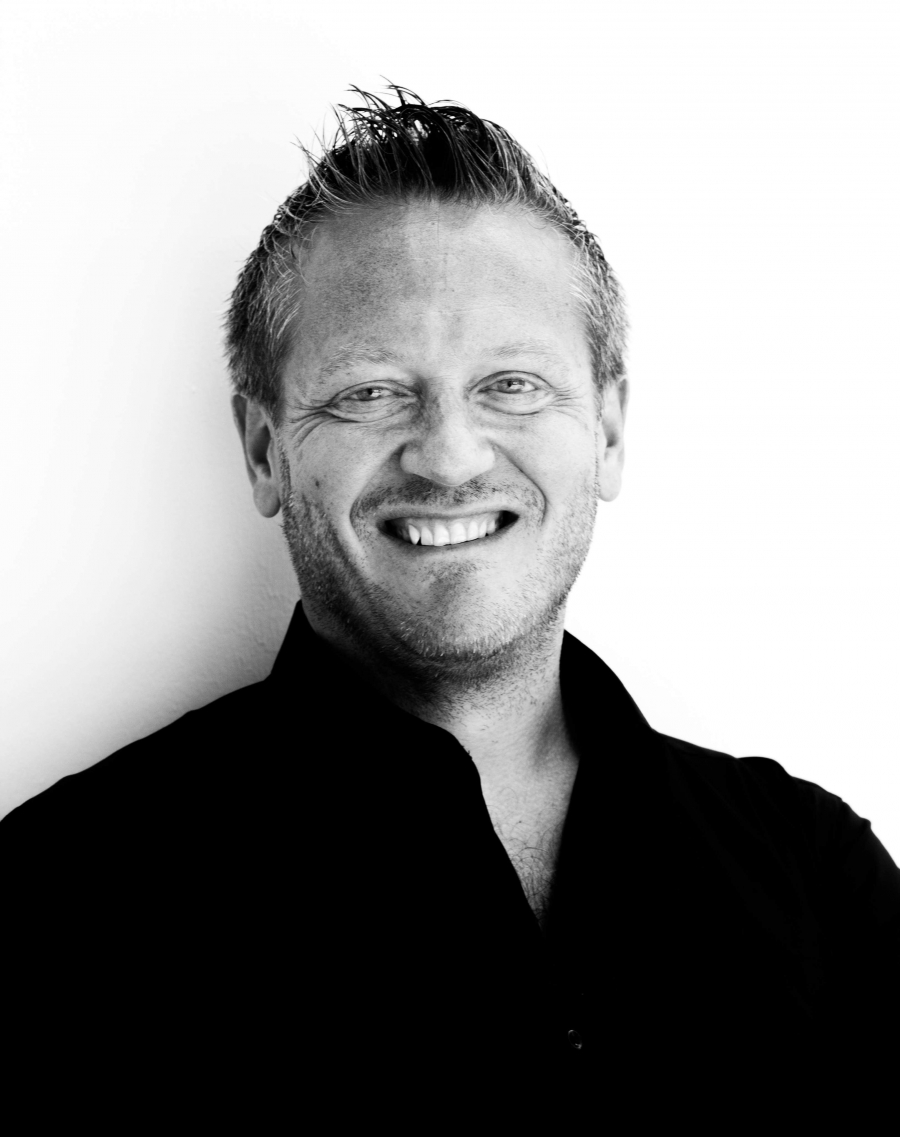
Working on trust and reliability of autonomous and intelligent systems
The second work package of the Astra project has a budget of € 3.1 million. This funding will support the creation of a state-of-the-art digital environment, not only for terrestrial technologies but also for the aerospace sector, as highlighted in previous articles.
A key aspect underpins this effort: reliability. This is the focus of the second task in WP2, aptly named "Trustworthiness of Smart and Autonomous Systems".
The introduction of machine learning and artificial intelligence (AI) technologies entails stringent reliability requirements. This is especially critical in the context of space applications, where the operating environment is inherently distinct from the familiar conditions on Earth.
Researchers at Astra are experimenting with integrating AI techniques into onboard software for of the Crystal Eye satellite. To this end, they are developing innovative methods to ensure that these intelligent and autonomous systems meet safety requirements and quality standards.
Addressing the reliability of AI systems is no trivial matter. "The work package I am responsible for is structured into several tasks, covering the digitalization of the production process,” explains Patrizio Pelliccione, Director of the Computer Science area at the Gran Sasso Science Institute. "This ranges from the development of onboard software platforms to the satellite’s digital architecture".
Building trust and reliability in autonomous space systems also involves refining the satellite's architecture. The research team is exploring how to implement "software watertight compartments" within the satellite, ensuring each component operates independently while contributing to the satellite's overall functionality. This approach aims to minimize potential issues and associated costs.
"Sometimes, the focus on AI is exaggerated", admits Professor Pelliccione. Currently, while AI can be applied to so-called "critical systems," the ultimate decision-making responsibility for critical events still resides with human engineers.
Critical systems have varying degrees of criticality depending on their controllability, which may be low (potentially leading to problematic or catastrophic impacts, as in the aerospace domain) or high, where controllability mitigates potential negative consequences. The reliability level, therefore, spans this spectrum.
“We are steadily moving towards a new era of AI, where it’s more controllable than in the past,” highlights Pelliccione. "High-quality software technology must not only be efficient and maintainable but must also adhere to explainability criteria".
Explainable AI (XAI) is a concept that has recently gained prominence in the field of machine learning. Its goal is to shed light on what occurs inside the “black box” of data and algorithms used to train AI models.
This topic extends beyond mere technological considerations, touching upon philosophical and ethical dimensions of AI. "Recently, I contributed to an article on how to engineer systems to truly serve humanity. While this goes beyond Astra's specific scope, it is crucial for our contributions—enabling satellite systems to articulate the rationale behind decisions made by AI", states Pelliccione.
Similarly, the recently enacted European AI Act emphasizes transparency, accountability, and explainability in automated actions. “When you deeply understand the reasoning behind an action, you begin to build trust between humans and machines,” concludes the professor.